This Paper:Browse 842 Download 34 |
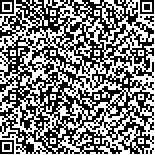 码上扫一扫! |
A neuro‑observer‑based optimal control for nonaffine nonlinear systems with control input saturations |
BehzadFarzanegan,MohsenZamani,AmirAbolfazlSuratgar,MohammadBagherMenhaj |
|
(Computational Intelligence Lab, Department of Electrical Engineering, Amirkabir University of Technology, Tehran, Iran;The School of Electrical Engineering and Computer Science, The University of Newcastle, Newcastle, Australia;Distributed Intelligent Optimization Research Lab, Department of Electrical Engineering, Amirkabir University of Technology, Tehran, Iran) |
|
摘要: |
|
关键词: |
DOI:https://doi.org/10.1007/s11768-021-00045-z |
|
基金项目: |
|
A neuro‑observer‑based optimal control for nonaffine nonlinear systems with control input saturations |
Behzad Farzanegan,Mohsen Zamani,Amir Abolfazl Suratgar,Mohammad Bagher Menhaj |
(Computational Intelligence Lab, Department of Electrical Engineering, Amirkabir University of Technology, Tehran, Iran;The School of Electrical Engineering and Computer Science, The University of Newcastle, Newcastle, Australia;Distributed Intelligent Optimization Research Lab, Department of Electrical Engineering, Amirkabir University of Technology, Tehran, Iran) |
Abstract: |
In this study, an adaptive neuro-observer-based optimal control (ANOPC) policy is introduced for unknown nonaffine
nonlinear systems with control input constraints. Hamilton–Jacobi–Bellman (HJB) framework is employed to minimize a
non-quadratic cost function corresponding to the constrained control input. ANOPC consists of both analytical and algebraic
parts. In the analytical part, first, an observer-based neural network (NN) approximates uncertain system dynamics,
and then another NN structure solves the HJB equation. In the algebraic part, the optimal control input that does not exceed
the saturation bounds is generated. The weights of two NNs associated with observer and controller are simultaneously
updated in an online manner. The ultimately uniformly boundedness (UUB) of all signals of the whole closed-loop system
is ensured through Lyapunov’s direct method. Finally, two numerical examples are provided to confirm the effectiveness of
the proposed control strategy. |
Key words: Input constraints · Optimal control · Neural networks · Nonaffine nonlinear systems · Reinforcement learning · Unknown dynamics |