This Paper:Browse 830 Download 11 |
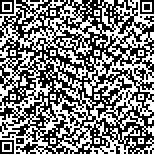 码上扫一扫! |
Neural-network-based stochastic linear quadratic optimal tracking control scheme for unknown discrete-time systems using adaptive dynamic programming |
XinChen,FangWang |
|
(1 School of Automation, China University of Geosciences, Wuhan 430074, Hubei, China
2 Hubei Key Laboratory of Advanced Control and Intelligent Automation for Complex Systems, Wuhan 430074, Hubei, China) |
|
摘要: |
|
关键词: |
DOI:https://doi.org/10.1007/s11768-021-00046-y |
|
基金项目:This work was supported by the National Natural Science Foundation of China (No. 61873248), the Hubei Provincial Natural Science Foundation of China (Nos. 2017CFA030, 2015CFA010), and the 111 project (No. B17040). |
|
Neural-network-based stochastic linear quadratic optimal tracking control scheme for unknown discrete-time systems using adaptive dynamic programming |
Xin Chen,Fang Wang |
(1 School of Automation, China University of Geosciences, Wuhan 430074, Hubei, China
2 Hubei Key Laboratory of Advanced Control and Intelligent Automation for Complex Systems, Wuhan 430074, Hubei, China) |
Abstract: |
In this paper, a stochastic linear quadratic optimal tracking scheme is proposed for unknown linear discrete-time (DT) systems
based on adaptive dynamic programming (ADP) algorithm. First, an augmented system composed of the original system
and the command generator is constructed and then an augmented stochastic algebraic equation is derived based on the
augmented system. Next, to obtain the optimal control strategy, the stochastic case is converted into the deterministic one
by system transformation, and then an ADP algorithm is proposed with convergence analysis. For the purpose of realizing
the ADP algorithm, three back propagation neural networks including model network, critic network and action network are
devised to guarantee unknown system model, optimal value function and optimal control strategy, respectively. Finally, the
obtained optimal control strategy is applied to the original stochastic system, and two simulations are provided to demonstrate
the effectiveness of the proposed algorithm. |
Key words: Stochastic system · Optimal tracking control · Adaptive dynamic programming · Neural networks |