This Paper:Browse 792 Download 10 |
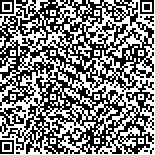 码上扫一扫! |
Adaptive Kalman filter for MEMS IMU data fusion using enhanced covariance scaling |
FuseiniMumuni,AlhassanMumuni |
|
(Electrical and Electronic Engineering Department, University of Mines and Technology (UMaT), P. O. Box 237, Tarkwa, Ghana;Electrical and Electronics Engineering Department, Cape Coast Technical University (CCTU), P. O. Box DL 50, Cape Coast, Ghana) |
|
摘要: |
|
关键词: |
DOI:https://doi.org/10.1007/s11768-021-00058-8 |
|
基金项目: |
|
Adaptive Kalman filter for MEMS IMU data fusion using enhanced covariance scaling |
Fuseini Mumuni,Alhassan Mumuni |
(Electrical and Electronic Engineering Department, University of Mines and Technology (UMaT), P. O. Box 237, Tarkwa, Ghana;Electrical and Electronics Engineering Department, Cape Coast Technical University (CCTU), P. O. Box DL 50, Cape Coast, Ghana) |
Abstract: |
MEMS (micro-electro-mechanical-system) IMU (inertial measurement unit) sensors are characteristically noisy and this
presents a serious problem to their effective use. The Kalman filter assumes zero-mean Gaussian process and measurement
noise variables, and then recursively computes optimal state estimates. However, establishing the exact noise statistics is a
non-trivial task. Additionally, this noise often varies widely in operation. Addressing this challenge is the focus of adaptive
Kalman filtering techniques. In the covariance scaling method, the process and measurement noise covariance matrices Q
and R are uniformly scaled by a scalar-quantity attenuating window. This study proposes a new approach where individual
elements of Q and R are scaled element-wise to ensure more granular adaptation of noise components and hence improve
accuracy. In addition, the scaling is performed over a smoothly decreasing window to balance aggressiveness of response
and stability in steady state. Experimental results show that the root mean square errors for both pith and roll axes are significantly
reduced compared to the conventional noise adaptation method, albeit at a slightly higher computational cost.
Specifically, the root mean square pitch errors are 1.1? under acceleration and 2.1? under rotation, which are significantly
less than the corresponding errors of the adaptive complementary filter and conventional covariance scaling-based adaptive
Kalman filter tested under the same conditions. |
Key words: IMU state-space model · Role and pitch estimation · More · Attitude estimation · Adaptive Kalman filter · Covariance scaling |