This Paper:Browse 652 Download 0 |
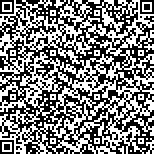 码上扫一扫! |
Stochastic model predictive braking control for heavy-duty commercial vehicles during uncertain brake pressure and road profile conditions |
RyotaNakahara1,KazumaSekiguchi1,KenichiroNonaka1,MasahiroTakasugi2,HirokiHasebe2,KenichiMatsubara2 |
|
(1 Graduate School of Integrative Science and Engineering, Tokyo City University, Tamazutsumi, Setagaya-ku, Tokyo 1588557, Japan;2 Nabtesco Automotive Co., Ltd., Hirakawacho, Chiyoda-ku, Tokyo 1020093, Japan) |
|
摘要: |
When heavy-duty commercial vehicles (HDCVs) must engage in emergency braking, uncertain conditions such as the brake
pressure and road profile variations will inevitably affect braking control. To minimize these uncertainties, we propose a
combined longitudinal and lateral controller method based on stochastic model predictive control (SMPC) that is achieved via
Chebyshev–Cantelli inequality. In our method, SMPC calculates braking control inputs based on a finite time prediction that
is achieved by solving stochastic programming elements, including chance constraints. To accomplish this, SMPC explicitly
describes the probabilistic uncertainties to be used when designing a robust control strategy. The main contribution of this
paper is the proposal of a braking control formulation that is robust against probabilistic friction circle uncertainty effects.
More specifically, the use of Chebyshev–Cantelli inequality suppresses road profile influences, which have characteristics
that are different from the Gaussian distribution, thereby improving both braking robustness and control performance against
statistical disturbances.Additionally, since theKalman filtering (KF) algorithm is used to obtain the expectation and covariance
used for calculating deterministic transformed chance constraints, the SMPC is reformulated as a KF embedded deterministic
MPC. Herein, the effectiveness of our proposed method is verified via a MATLAB/Simulink and TruckSim co-simulation. |
关键词: Heavy-duty commercial vehicle · Brake system · Stochastic model predictive control · Road profile |
DOI:https://doi.org/10.1007/s11768-022-00090-2 |
|
基金项目: |
|
Stochastic model predictive braking control for heavy-duty commercial vehicles during uncertain brake pressure and road profile conditions |
Ryota Nakahara1,Kazuma Sekiguchi1,Kenichiro Nonaka1,Masahiro Takasugi2,Hiroki Hasebe2,Kenichi Matsubara2 |
(1 Graduate School of Integrative Science and Engineering, Tokyo City University, Tamazutsumi, Setagaya-ku, Tokyo 1588557, Japan;2 Nabtesco Automotive Co., Ltd., Hirakawacho, Chiyoda-ku, Tokyo 1020093, Japan) |
Abstract: |
When heavy-duty commercial vehicles (HDCVs) must engage in emergency braking, uncertain conditions such as the brake
pressure and road profile variations will inevitably affect braking control. To minimize these uncertainties, we propose a
combined longitudinal and lateral controller method based on stochastic model predictive control (SMPC) that is achieved via
Chebyshev–Cantelli inequality. In our method, SMPC calculates braking control inputs based on a finite time prediction that
is achieved by solving stochastic programming elements, including chance constraints. To accomplish this, SMPC explicitly
describes the probabilistic uncertainties to be used when designing a robust control strategy. The main contribution of this
paper is the proposal of a braking control formulation that is robust against probabilistic friction circle uncertainty effects.
More specifically, the use of Chebyshev–Cantelli inequality suppresses road profile influences, which have characteristics
that are different from the Gaussian distribution, thereby improving both braking robustness and control performance against
statistical disturbances.Additionally, since theKalman filtering (KF) algorithm is used to obtain the expectation and covariance
used for calculating deterministic transformed chance constraints, the SMPC is reformulated as a KF embedded deterministic
MPC. Herein, the effectiveness of our proposed method is verified via a MATLAB/Simulink and TruckSim co-simulation. |
Key words: Heavy-duty commercial vehicle · Brake system · Stochastic model predictive control · Road profile |