This Paper:Browse 622 Download 0 |
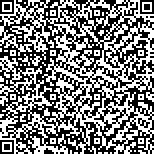 码上扫一扫! |
Development of stability-preserving time-limited model reduction framework for 2-D and 1-D models with error bound |
MuhammadImran1,SyedaHiraAmbreen2,SyedNoohHamdani2,MuhammadEjazNaveed2,MariaSiddiqui3 |
|
(1 Department of Electrical Engineering, Military College of Signals, (MCS), National University of Sciences and Technology (NUST), Islamabad 43000, Pakistan;2 Department of Electrical Engineering, Sir Syed (Center For Advanced Studies) Institute of Technology (SSCASE), Islamabad 43000, Pakistan;3 College of Science and Humanities, Rumah Majmaah University, Rumah, Kingdom of Saudi Arabia) |
|
摘要: |
Due to their complex structure, 2-Dmodels are challenging towork with; additionally, simulation, analysis, design, and control
get increasingly difficult as the order of the model grows. Moreover, in particular time intervals, Gawronski and Juang’s timelimited
model reduction schemes produce an unstable reduced-order model for the 2-D and 1-D models. Researchers revealed
some stability preservation solutions to address this key flaw which ensure the stability of 1-D reduced-order systems;
nevertheless, these strategies result in large approximation errors. However, to the best of the authors’ knowledge, there is
no literature available for the stability preserving time-limited-interval Gramian-based model reduction framework for the
2-D discrete-time systems. In this article, 2-D models are decomposed into two separate sub-models (i.e., two cascaded 1-D
models) using the condition of minimal rank-decomposition. Model reduction procedures are conducted on these obtained
two 1-D sub-models using limited-time Gramian. The suggestedmethodology works for both 2-D and 1-Dmodels. Moreover,
the suggested methodology gives the stability of the reduced model as well as a priori error-bound expressions for the 2-D and
1-D models. Numerical results and comparisons between existing and suggested methodologies are provided to demonstrate
the effectiveness of the suggested methodology. |
关键词: Model reduction · Weighted Gramian · Limited interval Gramian · Minimal realization · Approximation error · Error bound |
DOI:https://doi.org/10.1007/s11768-022-00109-8 |
|
基金项目: |
|
Development of stability-preserving time-limited model reduction framework for 2-D and 1-D models with error bound |
Muhammad Imran1,Syeda Hira Ambreen2,Syed Nooh Hamdani2,Muhammad Ejaz Naveed2,Maria Siddiqui3 |
(1 Department of Electrical Engineering, Military College of Signals, (MCS), National University of Sciences and Technology (NUST), Islamabad 43000, Pakistan;2 Department of Electrical Engineering, Sir Syed (Center For Advanced Studies) Institute of Technology (SSCASE), Islamabad 43000, Pakistan;3 College of Science and Humanities, Rumah Majmaah University, Rumah, Kingdom of Saudi Arabia) |
Abstract: |
Due to their complex structure, 2-Dmodels are challenging towork with; additionally, simulation, analysis, design, and control
get increasingly difficult as the order of the model grows. Moreover, in particular time intervals, Gawronski and Juang’s timelimited
model reduction schemes produce an unstable reduced-order model for the 2-D and 1-D models. Researchers revealed
some stability preservation solutions to address this key flaw which ensure the stability of 1-D reduced-order systems;
nevertheless, these strategies result in large approximation errors. However, to the best of the authors’ knowledge, there is
no literature available for the stability preserving time-limited-interval Gramian-based model reduction framework for the
2-D discrete-time systems. In this article, 2-D models are decomposed into two separate sub-models (i.e., two cascaded 1-D
models) using the condition of minimal rank-decomposition. Model reduction procedures are conducted on these obtained
two 1-D sub-models using limited-time Gramian. The suggestedmethodology works for both 2-D and 1-Dmodels. Moreover,
the suggested methodology gives the stability of the reduced model as well as a priori error-bound expressions for the 2-D and
1-D models. Numerical results and comparisons between existing and suggested methodologies are provided to demonstrate
the effectiveness of the suggested methodology. |
Key words: Model reduction · Weighted Gramian · Limited interval Gramian · Minimal realization · Approximation error · Error bound |