This Paper:Browse 768 Download 0 |
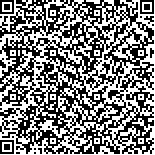 码上扫一扫! |
Distributed regularized online optimization using forward–backward splitting |
DemingYuan1,BaoyongZhang1,ShengyuanXu1,HuanyuZhao2 |
|
(1 School of Automation, Nanjing University of Science and Technology, Nanjing 210094, China;2 Faculty of Automation, Huaiyin Institute of Technology, Huai’an 223003, China) |
|
摘要: |
|
关键词: |
DOI:https://doi.org/10.1007/s11768-023-00134-1 |
|
基金项目:This work was supported in part by the National Natural Science Foundation of China (Nos. 62022042, 62273181 and 62073166), and in part by the Fundamental Research Funds for the Central Universities (No. 30919011105), and in part by the Open Project of the Key Laboratory of Advanced Perception and Intelligent Control of High-end Equipment (No. GDSC202017). |
|
Distributed regularized online optimization using forward–backward splitting |
Deming Yuan1,Baoyong Zhang1,Shengyuan Xu1,Huanyu Zhao2 |
(1 School of Automation, Nanjing University of Science and Technology, Nanjing 210094, China;2 Faculty of Automation, Huaiyin Institute of Technology, Huai’an 223003, China) |
Abstract: |
This paper considers the problem of distributed online regularized optimization over a network that consists of multiple interacting nodes. Each node is endowed with a sequence of loss functions that are time-varying and a regularization function that is fixed over time. A distributed forward–backward splitting algorithm is proposed for solving this problem and both fixed and adaptive learning rates are adopted. For both cases, we show that the regret upper bounds scale as O( √T ), where T is the time horizon. In particular, those rates match the centralized counterpart. Finally, we show the effectiveness of the proposed algorithms over an online distributed regularized linear regression problem. |
Key words: Distributed online optimization · Regularized online learning · Regret · Forward–backward splitting |