This Paper:Browse 802 Download 0 |
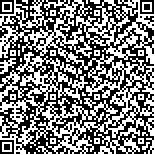 码上扫一扫! |
Automatic modeling algorithm of stochastic error for inertial sensors |
LuodiZhao1,2,3,LongZhao1,2,3 |
|
(1 School of Automation Science and Electrical Engineering, Beihang University, Beijing 100191, China;
2 Digital Navigation Center, Beihang University, Beijing 100191, China;
3 Science and Technology on Aircraft Control Laboratory, Beihang University, Beijing 100191, China.) |
|
摘要: |
This paper proposes an automatic algorithm to determine the properties of stochastic processes and their parameters for
inertial error. The proposed approach is based on a recently developed method called the generalized method of wavelet
moments (GMWM), whose estimator was proven to be consistent and asymptotically normally distributed. This algorithm
is suitable mainly (but not only) for the combination of several stochastic processes, where the model identification and
parameter estimation are quite difficult for the traditional methods, such as the Allan variance and the power spectral density
analysis. This algorithm further explores the complete stochastic error models and the candidate model ranking criterion to
realize automatic model identification and determination. The best model is selected by making the trade-off between the
model accuracy and the model complexity. The validation of this approach is verified by practical examples of model selection
for MEMS-IMUs (micro-electro-mechanical system inertial measurement units) in varying dynamic conditions. |
关键词: GMWM · Stochastic process · Inertial sensor · Sensor calibration · Error model · Allan variance |
DOI:https://doi.org/10.1007/s11768-023-00183-6 |
|
基金项目:This work was supported by the National Science Foundation of China (Nos. 42274037, 41874034), the Beijing Natural Science Foundation (No. 4202041), and the National Key Research and Development Program of China (No. 2020YFB0505804). |
|
Automatic modeling algorithm of stochastic error for inertial sensors |
Luodi Zhao1,2,3,Long Zhao1,2,3 |
(1 School of Automation Science and Electrical Engineering, Beihang University, Beijing 100191, China;
2 Digital Navigation Center, Beihang University, Beijing 100191, China;
3 Science and Technology on Aircraft Control Laboratory, Beihang University, Beijing 100191, China.) |
Abstract: |
This paper proposes an automatic algorithm to determine the properties of stochastic processes and their parameters for
inertial error. The proposed approach is based on a recently developed method called the generalized method of wavelet
moments (GMWM), whose estimator was proven to be consistent and asymptotically normally distributed. This algorithm
is suitable mainly (but not only) for the combination of several stochastic processes, where the model identification and
parameter estimation are quite difficult for the traditional methods, such as the Allan variance and the power spectral density
analysis. This algorithm further explores the complete stochastic error models and the candidate model ranking criterion to
realize automatic model identification and determination. The best model is selected by making the trade-off between the
model accuracy and the model complexity. The validation of this approach is verified by practical examples of model selection
for MEMS-IMUs (micro-electro-mechanical system inertial measurement units) in varying dynamic conditions. |
Key words: GMWM · Stochastic process · Inertial sensor · Sensor calibration · Error model · Allan variance |