This Paper:Browse 88 Download 0 |
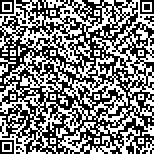 码上扫一扫! |
Data-based neural controls for an unknown continuous-time multi-input system with integral reinforcement |
YongfengLv1,JunZhao2,WanZhang1,HuiminChang3 |
|
(1 College of Electrical and Power Engineering, Taiyuan University of Technology, Taiyuan, Shanxi 030024, China;2 College of Transportation, Shandong University of Science and Technology, Qingdao 266590, Shandong, China;3 School of Mathematical Sciences, Shanxi University, Taiyuan 030006, Shanxi, China) |
|
摘要: |
Integral reinforcement learning (IRL) is an effective tool for solving optimal control problems of nonlinear systems, and it
has been widely utilized in optimal controller design for solving discrete-time nonlinearity. However, solving the Hamilton–
Jacobi–Bellman (HJB) equations for nonlinear systems requires precise and complicated dynamics. Moreover, the research
and application of IRL in continuous-time (CT) systems must be further improved. To develop the IRL of a CT nonlinear
system, a data-based adaptive neural dynamic programming (ANDP) method is proposed to investigate the optimal control
problem of uncertain CT multi-input systems such that the knowledge of the dynamics in the HJB equation is unnecessary.
First, the multi-input model is approximated using a neural network (NN), which can be utilized to design an integral
reinforcement signal. Subsequently, two criterion networks and one action network are constructed based on the integral
reinforcement signal. A nonzero-sum Nash equilibrium can be reached by learning the optimal strategies of the multi-input
model. In this scheme, the NN weights are constantly updated using an adaptive algorithm. The weight convergence and the
system stability are analyzed in detail. The optimal control problem of a multi-input nonlinear CT system is effectively solved
using the ANDP scheme, and the results are verified by a simulation study. |
关键词: Adaptive dynamic programming · Integral reinforcement · Neural networks · Heuristic dynamic programming · Multi-input system |
DOI:https://doi.org/10.1007/s11768-024-00238-2 |
|
基金项目: |
|
Data-based neural controls for an unknown continuous-time multi-input system with integral reinforcement |
Yongfeng Lv1,Jun Zhao2,Wan Zhang1,Huimin Chang3 |
(1 College of Electrical and Power Engineering, Taiyuan University of Technology, Taiyuan, Shanxi 030024, China;2 College of Transportation, Shandong University of Science and Technology, Qingdao 266590, Shandong, China;3 School of Mathematical Sciences, Shanxi University, Taiyuan 030006, Shanxi, China) |
Abstract: |
Integral reinforcement learning (IRL) is an effective tool for solving optimal control problems of nonlinear systems, and it
has been widely utilized in optimal controller design for solving discrete-time nonlinearity. However, solving the Hamilton–
Jacobi–Bellman (HJB) equations for nonlinear systems requires precise and complicated dynamics. Moreover, the research
and application of IRL in continuous-time (CT) systems must be further improved. To develop the IRL of a CT nonlinear
system, a data-based adaptive neural dynamic programming (ANDP) method is proposed to investigate the optimal control
problem of uncertain CT multi-input systems such that the knowledge of the dynamics in the HJB equation is unnecessary.
First, the multi-input model is approximated using a neural network (NN), which can be utilized to design an integral
reinforcement signal. Subsequently, two criterion networks and one action network are constructed based on the integral
reinforcement signal. A nonzero-sum Nash equilibrium can be reached by learning the optimal strategies of the multi-input
model. In this scheme, the NN weights are constantly updated using an adaptive algorithm. The weight convergence and the
system stability are analyzed in detail. The optimal control problem of a multi-input nonlinear CT system is effectively solved
using the ANDP scheme, and the results are verified by a simulation study. |
Key words: Adaptive dynamic programming · Integral reinforcement · Neural networks · Heuristic dynamic programming · Multi-input system |