This Paper:Browse 2121 Download 411 |
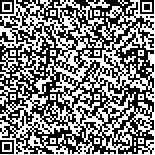 码上扫一扫! |
|
SilviaFerrari,JagannathanSarangapani,FrankL.Lewis |
|
(Laboratory for Intelligent Systems and Control (LISC), Department of Mechanical Engineering & Materials Science, Duke University;Department of Electrical & Computer Engineering, University of Missouri-Rolla;Automation and Robotics Research Institute, The University of Texas at Arlington) |
|
摘要: |
|
关键词: |
DOI: |
Received:May 18, 2011Revised:May 18, 2011 |
基金项目: |
|
Editorial: Special issue on approximate dynamic programming and reinforcement learning |
Silvia Ferrari,Jagannathan Sarangapani,Frank L. Lewis |
(Laboratory for Intelligent Systems and Control (LISC), Department of Mechanical Engineering & Materials Science, Duke University;Department of Electrical & Computer Engineering, University of Missouri-Rolla;Automation and Robotics Research Institute, The University of Texas at Arlington) |
Abstract: |
We are extremely pleased to present this special issue of the Journal of Control Theory and Applications. Approximate dynamic programming (ADP) is a general and effective approach for solving optimal control and estimation problems by adapting to uncertain environments over time. ADP optimizes the sensing objectives accrued over a future time interval with respect to an adaptive control law, conditioned on prior knowledge of the system, its state, and uncertainties. A numerical search over the present value of the control minimizes a Hamilton-Jacobi-Bellman (HJB) equation providing a basis for real-time, approximate optimal control. |
Key words: Special issue approximate dynamic programming (ADP) reinforcement learning |