This Paper:Browse 592 Download 0 |
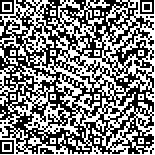 码上扫一扫! |
Robust state of charge and state of health estimation for batteries using a novel multi model approach |
GiovanniGuida1,DavideFaverato1,MarcoColabella1,GianlucaBuonomo1 |
|
(1 Innovation Department, Brain Technologies, Corso Tazzoli 215/12B, Turin 10137, Italy) |
|
摘要: |
Estimation of state-of-charge and state-of-health for batteries is one of the most important feature for modern battery management
system (BMS). Robust or adaptive methods are the most investigated because a more intelligent BMS could lead
to sensible cost reduction of the entire battery system. We propose a new robust method, called ERMES (extendible range
multi-model estimator), for determining an estimated state-of-charge (SoC), an estimated state-of-health (SoH) and a prediction
of uncertainty of the estimates (state-of-uncertainty—SoU), thanks to which it is possible to monitor the validity of the
estimates and adjust it, extending the robustness against a wider range of uncertainty, if necessary. Specifically, a finite number
of models in state-space form are considered starting from a modified Thevenin battery model. Each model is characterized
by a hypothesis of SoH value. An iterated extended Kalman filter (EKF) is then applied to each model in parallel, estimating
for each one the SoC state variable. Residual errors are then considered to fuse both the estimated SoC and SoH from the
bank of EKF, yielding the overall SoC and SoH estimates, respectively. In addition, a figure of uncertainty of such estimates
is also provided. |
关键词: Adaptive estimation multiple models · Connected embedded systems · Extended Kalman filter · Nonlinear observability · State-of-charge · State-of-health · State and parameter estimation |
DOI:https://doi.org/10.1007/s11768-022-00103-0 |
|
基金项目: |
|
Robust state of charge and state of health estimation for batteries using a novel multi model approach |
Giovanni Guida1,Davide Faverato1,Marco Colabella1,Gianluca Buonomo1 |
(1 Innovation Department, Brain Technologies, Corso Tazzoli 215/12B, Turin 10137, Italy) |
Abstract: |
Estimation of state-of-charge and state-of-health for batteries is one of the most important feature for modern battery management
system (BMS). Robust or adaptive methods are the most investigated because a more intelligent BMS could lead
to sensible cost reduction of the entire battery system. We propose a new robust method, called ERMES (extendible range
multi-model estimator), for determining an estimated state-of-charge (SoC), an estimated state-of-health (SoH) and a prediction
of uncertainty of the estimates (state-of-uncertainty—SoU), thanks to which it is possible to monitor the validity of the
estimates and adjust it, extending the robustness against a wider range of uncertainty, if necessary. Specifically, a finite number
of models in state-space form are considered starting from a modified Thevenin battery model. Each model is characterized
by a hypothesis of SoH value. An iterated extended Kalman filter (EKF) is then applied to each model in parallel, estimating
for each one the SoC state variable. Residual errors are then considered to fuse both the estimated SoC and SoH from the
bank of EKF, yielding the overall SoC and SoH estimates, respectively. In addition, a figure of uncertainty of such estimates
is also provided. |
Key words: Adaptive estimation multiple models · Connected embedded systems · Extended Kalman filter · Nonlinear observability · State-of-charge · State-of-health · State and parameter estimation |